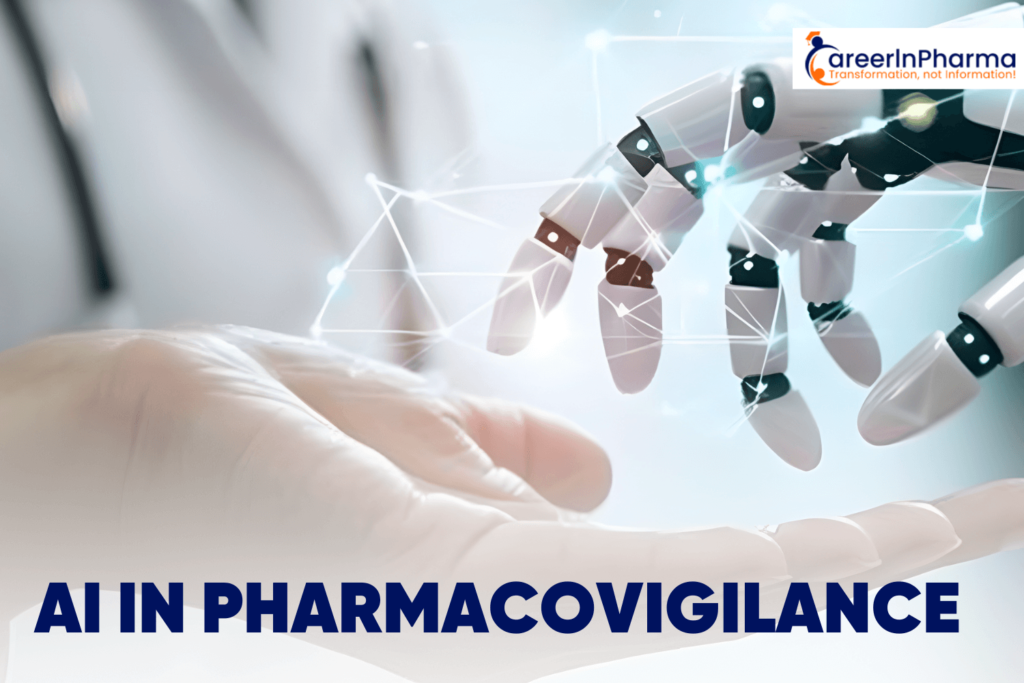
Pharmacovigilance ensures the safety and efficacy of any pharmaceutical drug products or medical devices throughout their lifecycle. Traditional methods available for monitoring Adverse Drug Reactions (ADRs) are resource-limited and prone to cause underreporting of ADRs. Recent technological advancement in pharmacovigilance reporting is the integration of artificial intelligence (AI) and ADR reporting.
AI in the Pharmacovigilance – Its Future
Artificial Intelligence (AI), automation, and analytics have emerged as evolving fields within pharmacovigilance (PV), accompanied by understandable concerns and questions. However, a closer examination reveals that many of these concerns stem from a limited understanding of these technologies and their potential impact on the pharmacovigilance industry.
AI, also known as machine intelligence, holds immense potential in the realm of Pharmacovigilance. With the massive amount of data involved in detecting, assessing, understanding, and preventing Adverse Drug Reactions (ADRs), the need for more healthcare professionals to handle and evaluate this data is evident. AI can significantly reduce the human workload, minimise errors, and ensure 100% compliance with regulatory authorities. For instance, the Pharmacovigilance Programme of India (PvPI) can benefit from automated input using AI techniques, reducing costs while advancing excellence and accuracy. By harmonising and monitoring collected data from different PV centres worldwide, AI-driven approaches can further advance drug safety. However, continuous research in the field of AI and its application in pharmacovigilance remains crucial. The development of AI, databases, and tools is currently at a prime stage, poised to shape the future of pharmacovigilance.
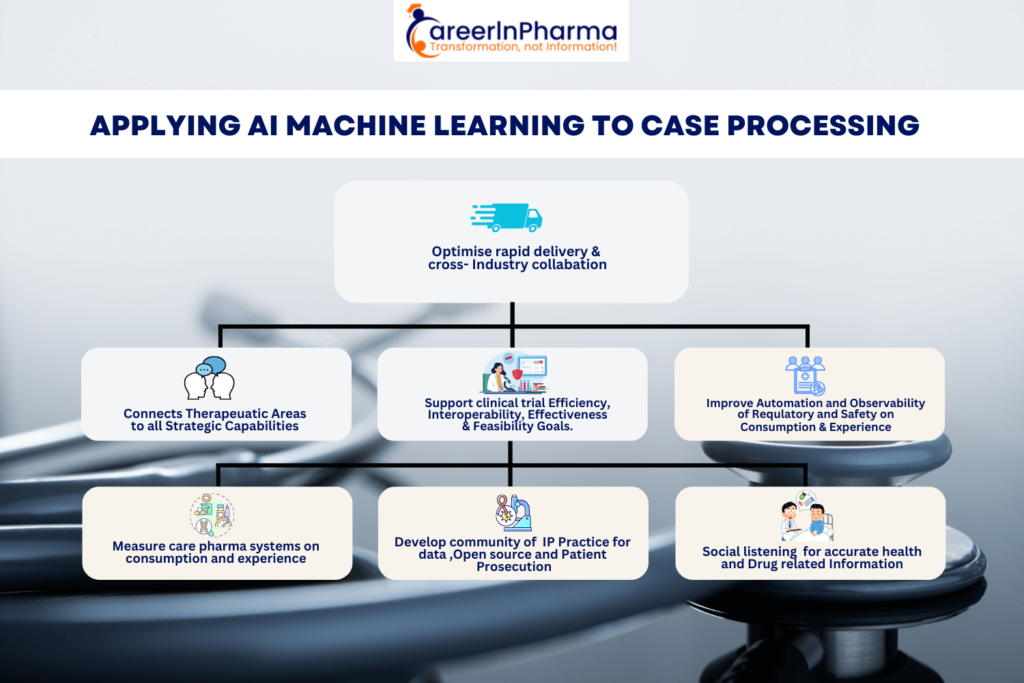
How AI Further Improves Pharmacovigilance
There are various factors other than just Adverse Events that define the extent of harm caused by a drug in official market-wide circulation. Some of these factors include:
- Risk Factor: This factor is referred to when the chances of adverse reactions to drugs become high.
- Adverse Drug Reaction (ADR): ADR refers to the adverse effects of drugs that occur when they are consumed as prescribed.
- Signal: When an initial causal relationship can be established between a drug and an adverse event, this information is known as a signal.
- Serious Reaction: When the adverse reaction to a drug is fatal, life-threatening, permanent disability, or congenital condition it is called a serious reaction.
These factors are the most important and sensitive sources of data that require intense scrutiny. Only the most granular and in-depth analysis of drug effects can lead to ideal patient outcomes from future versions of a specific drug that has formerly been labelled as harmful and that is exactly where AI can help. With the help of AI, the error rate can be decreased and the process of analyzing a tremendous amount of data worldwide can be simplified with great timeline benefits.
Automation Tools – Pharmacovigilance
AI-powered Automation tools for pharmacovigilance can recreate the way Adverse Drug Reactions (ADRs) are identified, reported, and managed. These tools use machine learning algorithms to analyze tremendous amounts of data from multiple sources, including social media, electronic health records and other forms of reliable and practical evidence.
The FDA’s Sentinel System is an example of such a tool which uses automated algorithms to analyze large healthcare databases to identify potential safety signals associated with medical and drug products.
IBM Watson is also an AI-powered Automation tool for Pharmacovigilance used for Drug Discovery, this tool uses machine learning and predictive analytics to accelerate drug discovery and development, It is also a data preparation and cleansing tool that can help identify and correct data quality issues in pharmacovigilance data sets.
Challenges of Using AI in Pharmacovigilance
Using AI in pharmacovigilance presents several challenges despite its potential to enhance drug safety monitoring and signal detection. Here are some key challenges:
1. Integration and Data Quality
- Heterogeneous Data Sources: Pharmacovigilance data comes from various sources that may be unstructured or formatted inconsistently.
- Data Completeness: Incomplete data or data with errors can lead to misleading insights and undermine the reliability of AI models.
2. Regulatory Compliance
- Lack of Clear Guidelines: Regulatory frameworks for AI use in pharmacovigilance are still evolving, making it challenging for organizations to ensure compliance.
- Validation Requirements: AI systems need validation to meet regulatory standards, which can be time-consuming and resource-intensive.
3. Signal Detection Complexity
- Contextual Understanding: AI might struggle to account for context or confounding factors that could influence adverse event reporting.
- False Positives: AI systems may generate false signals (indicating an adverse event that isn’t related to the drug) or miss real signals, complicating safety assessments.
4. Resource and Infrastructure Challenges
- High Implementation Costs: Developing, deploying, and maintaining AI systems can require significant investment in technology and talent.
5. Continuous Learning and Adaptation
- Model Updating: AI systems must be regularly updated with new data and information to remain effective, which can be challenging to manage.
- Dynamic Nature of Pharmacovigilance: The evolving nature of drug safety information requires AI systems to adapt quickly to new findings and regulations.
Addressing these challenges requires a collaborative effort among industry stakeholders, regulatory bodies, and technology providers to develop effective, reliable, and ethical AI solutions in pharmacovigilance.
Outcomes with AI are Better
By leveraging AI algorithms and machine learning techniques, pharmaceutical companies and regulatory agencies can ensure the safety of patients and improve public health outcomes. AI-powered pharmacovigilance offers several significant advantages over traditional methods, leading to improved outcomes.
- Faster Adverse Event Detection
- Improved Accuracy and Precision
- Cost Efficiency
- Regulatory Compliance and Reporting
- Personalized Drug Safety
- Real-Time Monitoring
- Improved Post-Market Surveillance
Conclusion
AI-powered pharmacovigilance offers several advantages over traditional methods, improves drug safety through faster detection, real-time monitoring, improved accuracy, and scalability. By processing huge amounts of data from diverse sources worldwide.
AI allows for earlier identification of possible risks and Adverse Drug Reactions. Its ability to integrate predictive analytics and personalized safety assessments ensures better patient outcomes, while also reducing human error and cost. AI improves the efficiency and effectiveness of pharmacovigilance, making it a superior tool for safeguarding public health.
Pharmacovigilance ensures the safety and efficacy of any pharmaceutical drug products or medical devices throughout their lifecycle. Traditional methods available for monitoring Adverse Drug Reactions (ADRs) are resource-limited and prone to cause underreporting of ADRs. Recent technological advancement in pharmacovigilance reporting is the integration of artificial intelligence (AI) and ADR reporting.
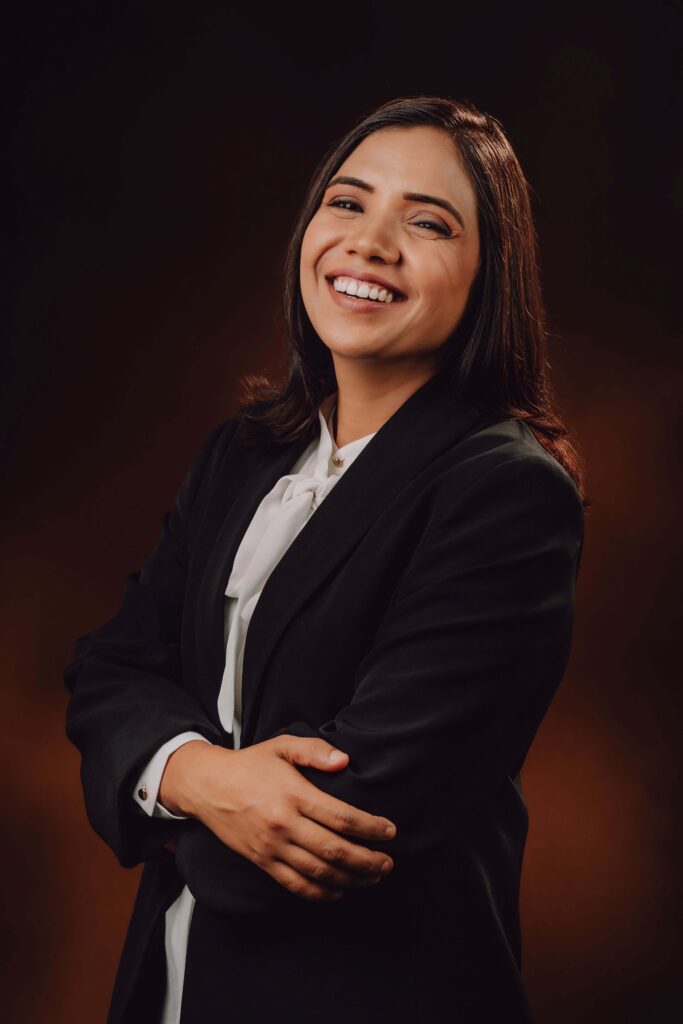